Why Traditional Market Research is Broken
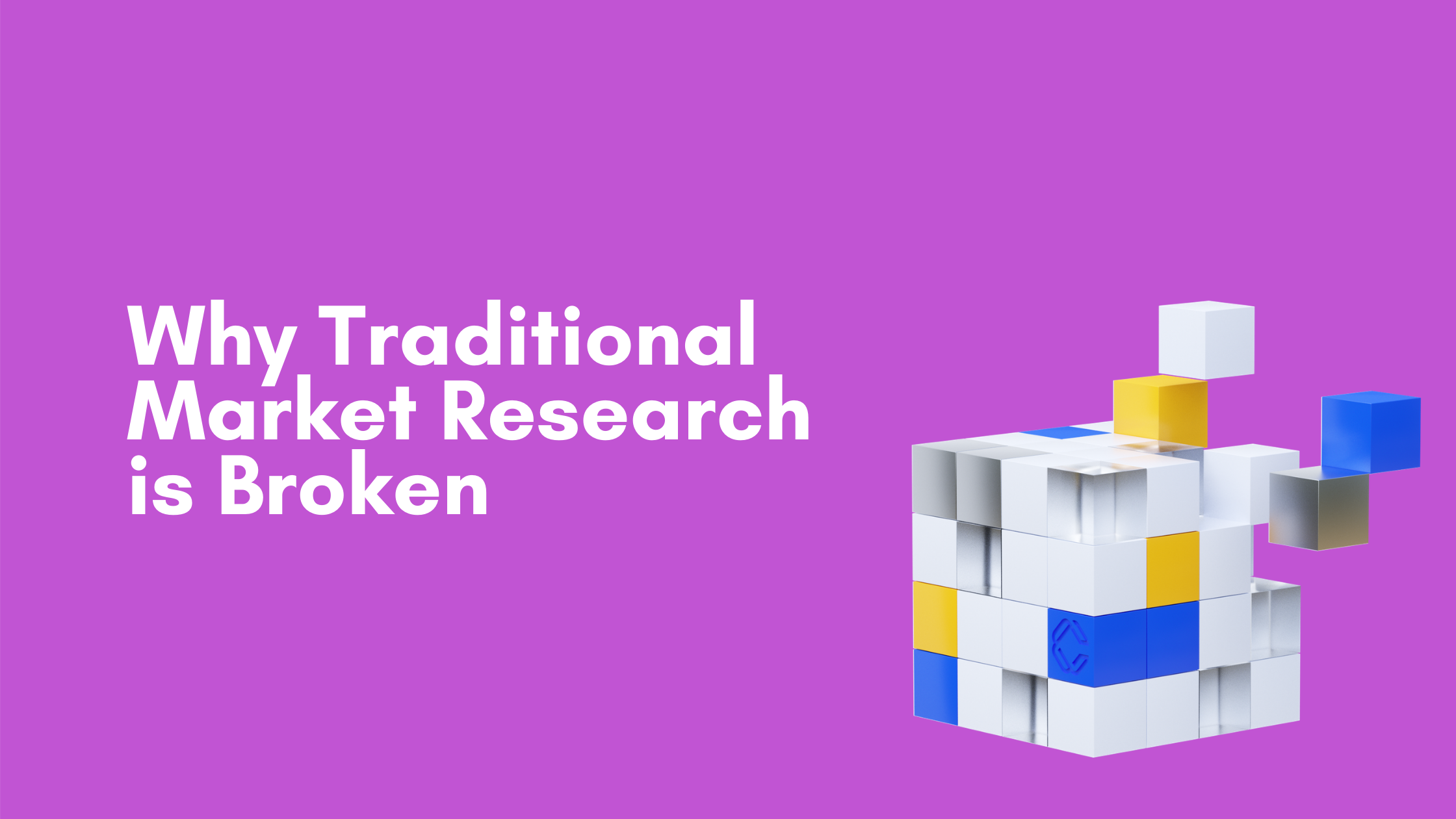
Steve Jobs is one of the greatest innovators of all time, from even far before the iPhone, iPod, Mac, and other modern electronics. A NeXT computer, created by Steve Jobs in his early years, was used to host the world’s first web server and was even used to write the first web browser.
In other words, Steve Jobs is a key authority for modern product innovators to learn from. At the same time, he has some advice that goes against the grain:
Some people say, "Give the customers what they want." But that's not my approach. Our job is to figure out what they're going to want before they do.
This sentiment, which has been echoed by some of the world's most respected CEOs and business leaders, is the impetus behind a new approach to conducting market research.
A new approach
With the rise in AI and big data, market research has evolved from relying on traditional surveys to a more advanced method that uses machine learning to predict what customers want for the future.
Traditionally, market research has been conducted through text surveys or focus groups. These methods have inherent flaws that make them inaccurate. For example, text surveys can only ask a finite number of questions, meaning that they are inevitably going to miss many important topics. Not to mention, text surveys - in contrast to voice surveys - are known to have incredibly low completion rates.
Focus groups are open to the influence of others in the group, leading to false answers. AI and big data offer more accuracy than these traditional methods, by extracting insights from the source of customer conversations online.
Instead of asking their customers what they want with tedious text surveys, companies are now relying on algorithms. Commerce.AI identifies trends in customer behaviour, which makes it an excellent tool for predicting customer needs based on what they've done in the past. Companies use this data to formulate strategies for specific products, services, and features before even developing them.
By abandoning old-school manual survey data crunching for AI and big data, businesses are able to make better decisions about their products and services before investing time and resources into them. This new form of market research allows businesses to be more responsive to changing customer needs and leads to more innovative products and services.
Being demand-driven
There are many factors influencing the success of a new product.
One of the most important, and often surprisingly overlooked, is understanding the concept of demand. You always need to know where the demand is, and build your product around it.
Many people make the mistake of building a product without considering how to make potential customers want it. They create something because they think it's a good idea, or because they think they can sell it at a profit.
This approach doesn't work for two reasons. First, you don't know whether there are enough customers out there who want your product, so you're wasting your time and money if you invest in marketing and expansion before you have solid data. Second, even if there are customers who want your product, they may not be willing to pay what you need to charge in order to make a profit on each sale.
Instead of trying to create demand for your products after you've created them, build in-demand products and features instead. This means developing a product that satisfies a demand for an unmet need, which can be revealed by Commerce.AI's data engine that scans billions of data points across the Internet.
Once you've done this, all you need to do is market your solution as the best way to meet that need—and focus on making it really easy for potential customers to buy it.
Understanding product demand with AI
Many product companies have a hard time predicting product demand trends because they don't have the data analysts or time to manually analyze live data at scale. This is where predictive analytics come in - a machine learning technique that uses historical data to make predictions.
Machine Learning is not perfect, but it is a lot better than what many product firms are doing right now - which is guessing. Predictive analytics helps businesses predict what products will sell before they actually create and market the product. This allows them to accurately assign tasks to their product teams, allocate their inventory, and minimize losses, leading to increased revenue as well as better customer service.